How will the lag between innovation and regulation affect AI?
Death to humanity, speeding up hardware development, domain specific LLMs, climate change pressures, legal issues in AI
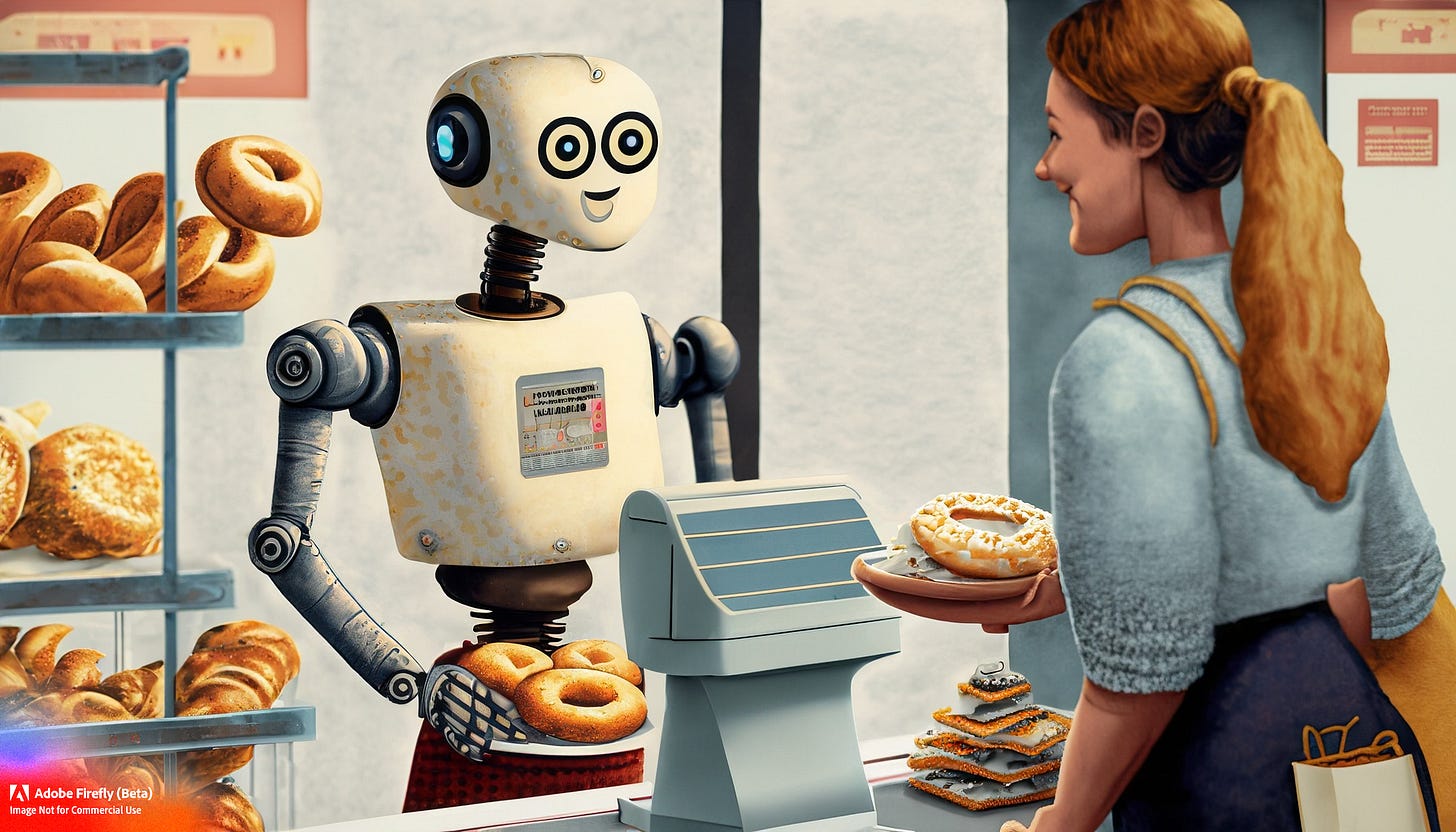
Social
To kick things off let’s look at social dynamics: In an Time magazine Op-Ed, AI pioneer Eliezer Yudkowsky argues that the recent letter calling for a 6-month pause on powerful AI systems is not enough, but that all development should stop. The basic argument is we are incapable of understanding if they are self-aware, or if it will be concerned about other sentient life. We simply aren’t prepared to get artificial general intelligence right on the first try, and if we don’t he argues the outcome is the death of all humans.
What’s changing: Opposition to AI and concerns about digital overloads is not new, it’s a sci-fi trope going back decades and sits in the middle of many Hollywood hits. Now, the concerns are coming from vocal but knowledgable experts and are getting put more in the social awareness. This article is in a major US magazine that generally publishes high quality content, and proposes complete shut down of all AI development.
What’s possible: There are a lot of implications that may arise. If the narrative of ‘shut it down’ becomes common, it could permeate political races and pundit circles. It could lead to protesting those responsible (hardware, software, research, anyone who uses AI). It could lead to asymmetric responses where some nations or companies halt but others continue. The assumption that AI leads to death of humanity is pretty stark and difficult to prove, so the thing to watch here is sentiment.
Technological
Next up, technology: NVIDIA announced the ability to improve the speed and power consumption of inverse lithography design by 40x (a step in making chips which a software program designs a critical piece for chip production that requires a massive hardware stack and weeks). This isn’t just running on a GPU over a CPU, this is optimizing the processing specifically for GPUs.
What’s changing: As chips approach the laws of physics, software increasingly plays a role in helping to develop advancements and make the big improvements expected to keep pace of miniaturization and cost reduction expected. NVIDIA is working with foundries to allow for faster, cheaper, less carbon intensive development of chips, which of course adds value. It also shows the value of massive parallelization of work that GPUs excel at, and how more specific use cases can be significantly improved if the right attention is placed.
What’s possible: Look for more opportunities to reduce costs, improve quality, and other powerful transformations that can come from hardware improvements. Training AI has always been better suited for GPUs, but what other opportunities (with the right technical approach) could be improved?
Economic
In the economic category: Bloomberg has trained its own large language model (LLM) specifically on finance related content. This is detailed in a research paper, if you are interested in the technical details about the 50 billion parameters from a 363 billion token dataset.
What’s changed: There are multiple LLMs out there today, but so far they are mostly general purpose. This is an LLM dedicated specifically to the financial domain (though it almost certainly will have some generalized capabilities). To my knowledge, this is the first LLM of this size dedicated to a single domain. Given its development by a major financial firm, this indicates interest in fully developing custom LLMs for more narrow applications rather than building on other’s systems.
What’s possible: There are a few factors at play here, the cost to develop a custom LLM is not out of reach for many large organizations if they have the technical interest and allocate the budget. The knowledge, tools, and awareness of how to develop an LLM are spreading, so I would expect to see more attempts at this. There are a lot of business models expecting that most organizations will want to customize an existing system instead of developing one in house, which still seems safe for today but need to watch the cost factors, tools, and data requirements at play.
Environmental
Next up, the environment: Vanuatu, a Pacific island nation of approximately 300k, has submitted a United Nations resolution “that should make it easier to hold polluting countries legally accountable for failure to act on the climate crisis.” The island nation has recently had two category four hurricanes strike within days of one another, and is at high risk of rising sea levels.
What’s changing: While not specifically about AI, this is potentially a major step towards increased international pressure to not only limit their climate impacts but also be financially assessed by what harm they are causing to other nations. While international courts have limited impact, they are potentially binding in some nations who ratify certain treaties.
What’s possible: This could be an avenue for future pressure to be placed on AI development, so its worth watching how this plays out. Also, data centers and technology are a prime target for reduction of climate impact. Carbon offsets are likely not going to be a shield forever (despite their shaky track record), and the best approach is actual reduction. Power and carbon conscious systems may get a boost.
Political
Last category is politics: Stable Diffusion is currently the target of several lawsuits, and the lawsuits are generally about the rights of images used in training data. There are arguments on both sides, but the key thing I want to focus on is the frame of the arguments since that is what will likely lead to a ruling. Certain prompts can generate the nearly identical outputs to some of the source materials (in this case images), and it is unclear how to handle the disagreement between parties about this result. It is not the same technical image nor is it directly copied, but it is generated.
What’s changing: Software rights and asset licensing are being put to the test, and until these lawsuits progress further its unclear where they might go. This could be landmark court cases, or they could settle out of court which would only defer the question for another time. Systems likely need to find ways to ensure they are doing their diligence and properly auditing the content going into their systems to minimize exposure, but a legal precedent might set more comprehensive limits or barriers.
What’s possible: A likely outcome here is the legal system tries to stabilize by leaning on past understandings of rights and usage, which could lead to major barriers for developing the massive datasets currently being used to train AI systems. If rights to all materials is required, it also opens up questions about possible licensing costs and the ability to better audit that the source materials aren’t copied.
This article is full of interesting and thought provoking ideas. AI is moving so quickly!